ML Engineer vs. Data Scientist: What’s the Difference?
There is a lot of confusion surrounding the role of a machine learning engineer and data scientist. Some people think they are one and the same, while others believe they are in completely different positions. So, what is the difference?
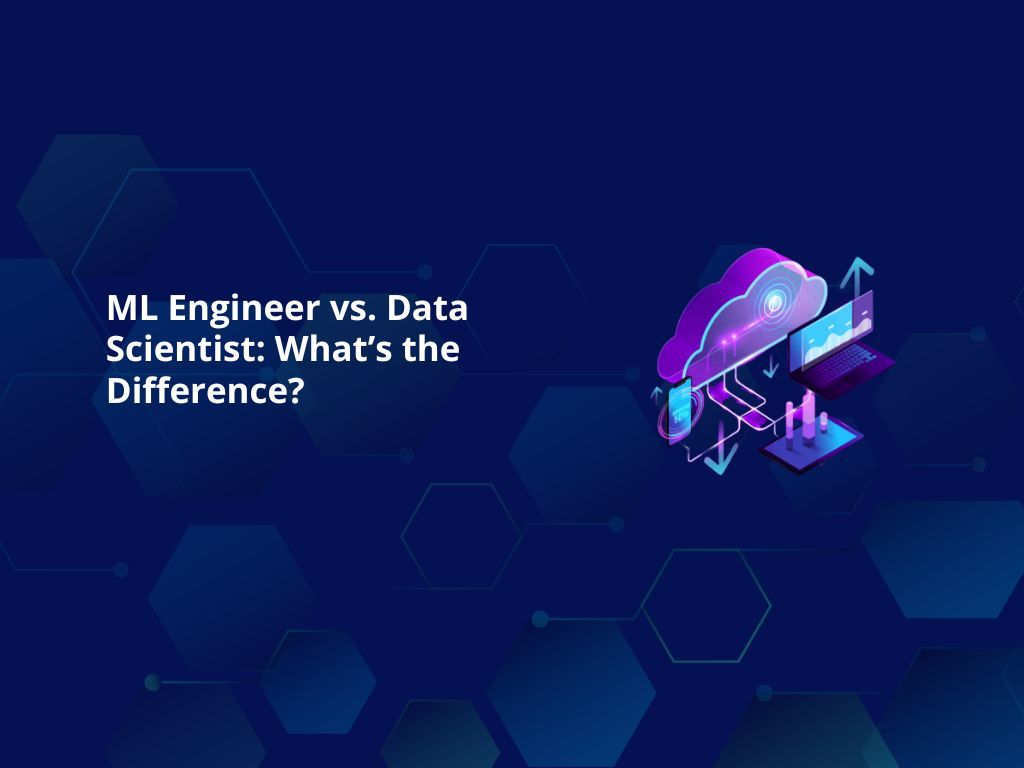
Artificial intelligence solutions to implement within your organization require you to understand the distinctions between these two roles.
There is a lot of confusion surrounding the role of a machine learning engineer and data scientist. Some people think they are one and the same, while others believe they are in completely different positions. So, what is the difference? And when do the role of a data scientist end and machine learning engineer begins? In this blog post, we will clear up any confusion and discuss the core steps in executing a successful data science or machine learning project. But let's look in detail at what these concepts cover.
Data Scientist vs Machine Learning Engineer
A Machine Learning (ML) Engineer is a computer scientist who designs and develops ML algorithms for machines to learn from data. This requires the engineer to understand machine learning fundamentals, such as supervised and unsupervised learning, linear regression, artificial neural networks, etc. There are also software engineering skills involved in building scalable ML models that can be used for production.
A Data Scientist is a specialist who collects, cleans, and processes data in order to derive insights from it. This requires not only an understanding of statistics and data mining but also software engineering skills to build data pipelines. They are responsible for identifying trends and patterns from data, as well as creating visualizations that make the information digestible to stakeholders.
So, when do the role of a data scientist end and machine learning engineer begin? Generally speaking, a data scientist will pre-process the data, make sense of it and create predictive models that can be used to generate insights. The ML engineer will then take those models and build algorithms that can be used in production.
The core steps in executing a successful data science and machine learning project include data collection, exploration, and cleaning, feature engineering, model building, validation, evaluation, and deployment. The role of the ML engineer is to take models created by the data scientist, build efficient ML algorithms that can be used on new data and deploy them in production in a scalable way.
The roles of data scientist and machine learning engineer might seem similar, but each position plays an essential role in executing successful ML projects. Data scientists are responsible for preparing the data, understanding patterns, and generating predictive models; while machine learning engineers build efficient algorithms that can be used for production. Together, they make up the perfect team to create a successful machine-learning project.
But what makes the two techniques different?
While data science is primarily focused on understanding and interpreting data, machine learning focuses on creating algorithms that can be used to make predictions based on the data. Data science is a more comprehensive approach, while machine learning is more specialized.
Careers Opportunities with Machine Learning and Data Science
Whether you’re interested in data science or machine learning, there are plenty of career opportunities in the field. Machine learning engineers and data scientists are both in high demand, and companies are looking for candidates that have experience with both disciplines. If you have a strong understanding of machine learning fundamentals as well as software engineering skills, then you can make a great machine learning engineer. And if you have an understanding of statistics, data mining and software engineering, then you can make a great data scientist.
How do you choose between Data Science and Machine Learning?
That’s it for our data science vs machine learning comparison. The fact is you cannot choose only one. Both data science and machine learning go hand in hand. In the future, data scientists will need at least a basic understanding of machine learning to model and interpret big data that is generated every single day.
If you are just starting your career or are from different backgrounds like Java or .NET, there is nothing to worry about.
Data Science is vast but not difficult. Since it has many stages, a data scientist’s job is divided into different sub-fields.
What technique do I need to choose for my project?
It all depends on the kind of problem you are trying to solve. Do you want to make predictions based on data or do you want to analyze and interpret the data? If you are looking for a predictive solution, then machine learning is the way to go. If you are looking for more explanatory insights from your data, then data science could be the right choice. Ultimately, it is up to you and your team's expertise.
Whatever technique you choose, remember that successful projects require both data scientists and machine learning engineers to work together as a cohesive unit.
In conclusion, it can be seen that the roles of data scientists and machine learning engineers are distinct but related. Data scientists are responsible for preparing the data, understanding patterns, and creating predictive models while machine learning engineers build efficient algorithms that can be used in production. Working together, they form a powerful team to execute successful machine learning projects. Lexunit can help you with your data science and ML projects, so contact us today to learn more about our services.
Good luck with your journey in Data Science!
We hope this article helped you to understand the differences between Machine Learning Engineer and Data Scientist roles. As you can see, they both play an important role in executing successful ML projects, but their approaches are slightly different. It's also important to note that machine learning and data science go hand in hand, so having knowledge of both will help you become a better data scientist or engineer. We at Lexunit wish you success on your journey!