Predictive forecasting models & Machine Learning
Anyone running a business wants to predict its future. Though it is not possible exactly to some extent, you can get an estimation because business comes with uncertainty and risks that can not be avoided but can be minimized using Predictive Forecasting.
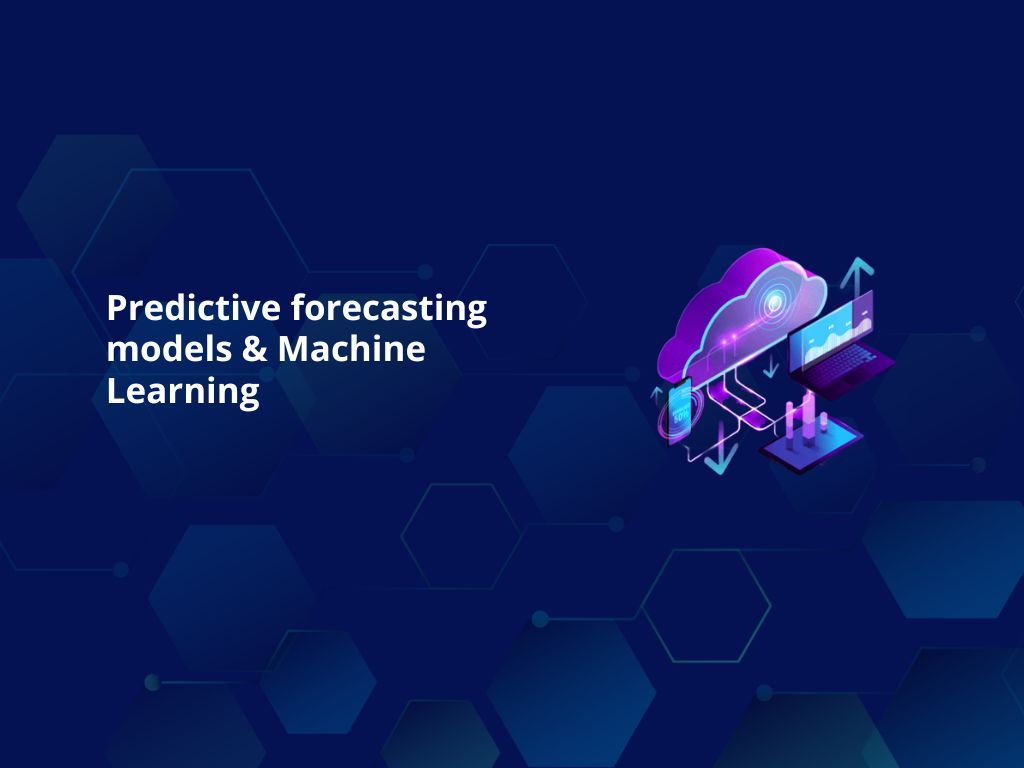
Anyone running a business wants to predict its future. Though it is not possible exactly to some extent, you can get an estimation because business comes with uncertainty and risks that can not be avoided but can be minimized using Predictive Forecasting.
But what is predictive forecasting?
Predictive forecasting is a predictive analytics technique used to forecast future outcomes and trends. Predictive forecasting models use historical data, statistical analysis, and machine learning algorithms to make predictions about the future. This type of predictive modeling can be used in a range of business sectors including finance, marketing, economics, healthcare, and retail.
Forecasting is the process of making predictive decisions by predicting future events and trends. Forecasting relies on analyzing historical data to identify patterns and draw conclusions about the future. Predictive forecasting is a more advanced technique that applies predictive machine learning algorithms to forecast future outcomes with greater detail and accuracy than traditional forecasting methods.
The predictive forecasting models use machine learning algorithms such as neural networks and support vector machines to learn from historical data, identify patterns and trends, and make predictions about future events. These predictive models are used in a range of business sectors including finance, marketing, economics, healthcare, and retail.
Using predictive forecasting with machine learning can provide businesses with valuable insights into the future which can drive decisions about strategy, product development roadmaps, competitive positioning, customer service plans, and more. Predictive forecasting is becoming increasingly important for businesses that want to stay one step ahead of their competition by leveraging the power of predictive analytics.
Top 5 Types of Predictive Models
Fortunately, predictive models don’t have to be created from scratch for every application. Predictive analytics tools use a variety of vetted models and algorithms that can be applied to a wide spread of use cases.
Predictive modeling techniques have been perfected over time. As we add more data, more muscular computing, AI, and machine learning and see overall advancements in analytics, we’re able to do more with these models.
The top five predictive analytics models are:
- Classification model: Considered the simplest model, it categorizes data for simple and direct query responses. An example use case would be to answer the question “Is this a fraudulent transaction?”
- Clustering model: This model nests data together by common attributes. It works by grouping things or people with shared characteristics or behaviors and planning strategies for each group at a larger scale. An example is in determining credit risk for a loan applicant based on what other people in the same or a similar situation did in the past.
- Forecast model: This is a very popular model, and it works on anything with a numerical value based on learning from historical data. For example, in answering how much lettuce a restaurant should order next week or how many calls a customer support agent should be able to handle per day or week, the system looks back to historical data.
- Outliers model: This model works by analyzing abnormal or outlying data points. For example, a bank might use an outlier model to identify fraud by asking whether a transaction is outside of the customer’s normal buying habits or whether an expense in a given category is normal or not. For example, a $1,000 credit card charge for a washer and dryer in the cardholder’s preferred big box store would not be alarming, but $1,000 spent on designer clothing in a location where the customer has never charged other items might be indicative of a breached account.
- Time series model: This model evaluates a sequence of data points based on time. For example, the number of stroke patients admitted to the hospital in the last four months is used to predict how many patients the hospital might expect to admit next week, next month, or the rest of the year. A single metric measured and compared over time is thus more meaningful than a simple average.
Predictive forecasting in Machine Learning projects offers predictive modeling capabilities to help businesses make decisions and plan for the future. By leveraging predictive models, businesses can anticipate customer behavior, manage inventory more efficiently, identify potential risks and opportunities early on and gain a competitive advantage.
Challenges of Predictive Modeling
It’s essential to keep predictive analytics focused on producing useful business insights because not everything this technology digs up is useful. Some mined information is of value only in satisfying a curious mind and has few or no business implications. Getting side-tracked is a distraction few businesses can afford.
Also, being able to use more data in predictive modeling is an advantage only to a point. Too much data can skew the calculation and lead to a meaningless or erroneous outcome. For example, more coats are sold as the outside temperature drops. But only to a point. People do not buy more coats when it’s -20 degrees Fahrenheit outside than they do when it’s -5 degrees below freezing. At a certain point, cold is cold enough to spur the purchase of coats, and more frigid temps no longer appreciably change that pattern.
And with the massive volumes of data involved in predictive modeling, maintaining security and privacy will also be a challenge. Further challenges rest in machine learning’s limitations.
The Future of Predictive Modeling
Predictive modeling, also known as predictive analytics, and machine learning are still young and developing technology, meaning there is much more to come. As techniques, methods, tools, and technologies improve, so will the benefits to businesses and societies.
However, these are not technologies that businesses can afford to adopt later after the tech reaches maturity and all the kinks are worked out. The near-term advantages are simply too strong for a late adopter to overcome and remain competitive. For further reading on broader AI adaption, check our 'The AI Journey' site.
Our advice: Understand and deploy the technology now and then grow the business benefits alongside subsequent advances in the technologies.
Lexunit is an AI development agency that can help you embrace predictive forecasting. With our predictive models, businesses have the opportunity to anticipate customer behavior, manage inventory more efficiently, identify potential risks and opportunities early on and gain a competitive advantage. Contact us today to learn more!
Predictive forecasting is a powerful tool for businesses seeking success in a turbulent world. By leveraging predictive models using machine learning algorithms, businesses can stay ahead of the competition and make decisions based on data-driven insights. As predictive modeling matures, its value will only increase – making it an essential part of any successful business strategy.
Lexunit’s team of AI experts is ready to help you get the most out of predictive forecasting so your organization can stay ahead of the curve. Let’s get started on predictive forecasting today!