Unleashing the Power of Data Augmentation to Tackle Climate Challenges for Startups
Climate-Tech startups often grapple with data limitations. Data augmentation can magnify and diversify their datasets, leading to robust, accurate AI models. With expert guidance, they can transform climate challenges into innovation opportunities.
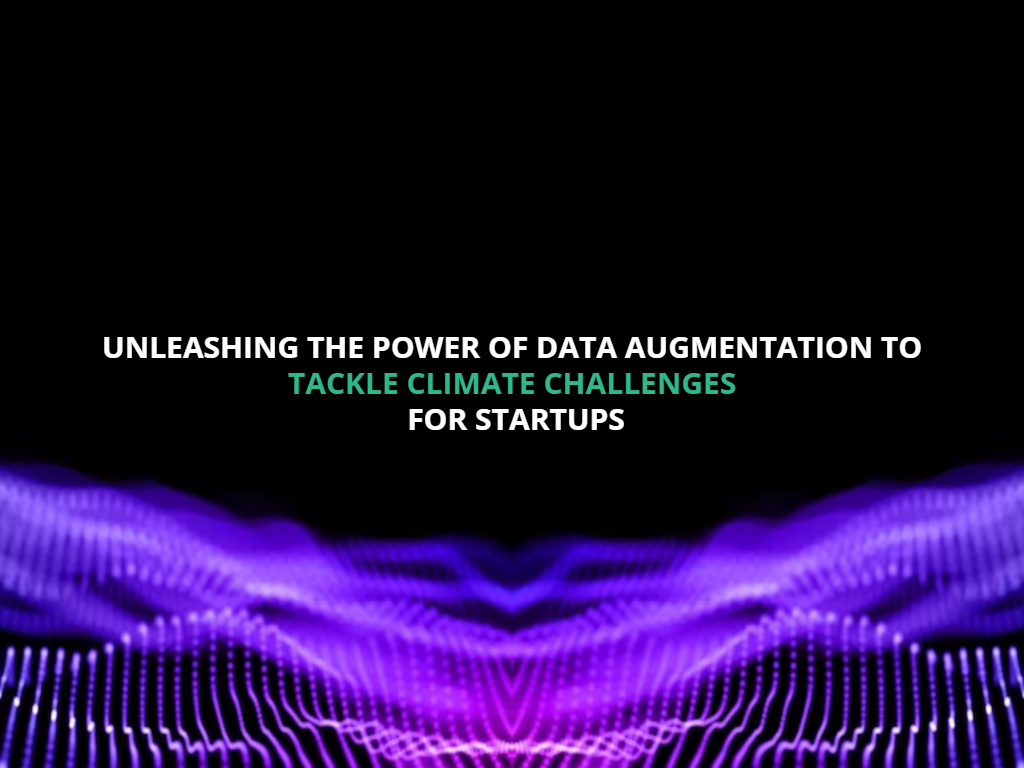
At Lexunit we are passionate about the topic of machine learning and its use cases to tackle climate change, but without the necessary amount of data, everything gets complicated.
Climate change is an urgent, existential threat facing humanity. According to a report by the Intergovernmental Panel on Climate Change (IPCC), it's more crucial than ever for nations to take drastic measures to curb greenhouse gas emissions. This opens an immense window of opportunity for startups in the Climate-Tech sector to innovate and drive sustainable solutions. However, these startups often face a significant obstacle: the lack of sufficient and robust data, which can impede the development and deployment of AI-powered solutions. Fortunately, there's a solution: data augmentation.
Understanding the Power of Data Augmentation
Data augmentation, as discussed in this research paper, is a technique employed to expand and diversify data samples. In simpler terms, it generates more data from existing labeled datasets, a practice that can prove vital when the available data is limited.
In the context of climate-tech startups, this can mean the ability to improve climate prediction models, carbon footprint calculators, or disaster management systems by enhancing the available data. This technique not only makes models more robust and accurate but also prevents overfitting on limited or small datasets.
Why Data Augmentation Matters for Climate-Tech Startups
Accurate data is the lifeblood of climate science. It’s needed to analyze climate change patterns, predict future trends, and create strategies to mitigate adverse impacts. However, it’s not always easy to collect this data, especially in the vast and varied field of climate science. Here's where data augmentation can significantly help.
Using data augmentation techniques, startups can enrich their datasets, improving accuracy and reducing overfitting. By understanding and implementing appropriate transformations on existing data, startups can enhance the data’s representativeness, providing robustness to the predictive models.
Implementing Data Augmentation in Climate-Tech
Consider a startup focused on developing an AI model to predict wildfires. They have historical wildfire data, but it's limited and doesn't fully capture the complexity of different wildfire scenarios. Using data augmentation, they could create a broader range of wildfire scenarios by tweaking the existing data. Techniques such as changing brightness and contrast or adding noise to images could simulate different lighting conditions and perspectives.
The same principle applies to other areas within climate-tech. For example, startups working on flood prediction can manipulate existing data to better represent various flood intensities and environments. Or those working on renewable energy prediction models can augment existing weather data to create more diverse scenarios, enhancing their models' ability to predict energy production under different weather conditions.
Data Augmentation Challenges and Overcoming Them
While promising, data augmentation isn't a silver bullet. Some limitations include ensuring the quality of augmented datasets, building synthetic data with advanced applications, and verifying image augmentation techniques like GANs. Another challenge lies in selecting the right augmentation strategy for the data, which can be non-trivial. Lastly, the inherent bias of original data can persist in the augmented data.
However, with a careful approach, these challenges can be overcome. Companies like Lexunit offer MLOPS services that can help startups understand their datasets and employ effective data augmentation techniques for their deep learning models. With their expertise and experienced team, they can help your business create robust deep-learning models with high accuracy.
Wrapping Up
The climate crisis is complex, and tackling it requires robust and accurate data models. As climate-tech startups continue to innovate in this space, the use of data augmentation can enhance their datasets, build more accurate models, and ultimately, deliver more impactful solutions.
With careful implementation and expert guidance, startups can leverage data augmentation to not just overcome data scarcity but also transform the climate crisis into an opportunity for impactful innovation.
Sources:
- IPCC Climate Change 2021 Report
- Alomar K, Aysel HI, Cai X. Data Augmentation in Classification and Segmentation: A Survey and New Strategies. J Imaging. 2023 Feb 17;9(2):46. doi: 10.3390/jimaging9020046. PMID: 36826965; PMCID: PMC9966095.