Optimizing automatic warehouse storage control
About the project
We collaborated with AtlasCopco to optimize their tray system that serves parts required for the day’s work. This enabled higher efficiency and lower maintenance costs.
Location:
Hungary
Industry:
Automotive
Services:
Data analytics
Business type:
Enterprise
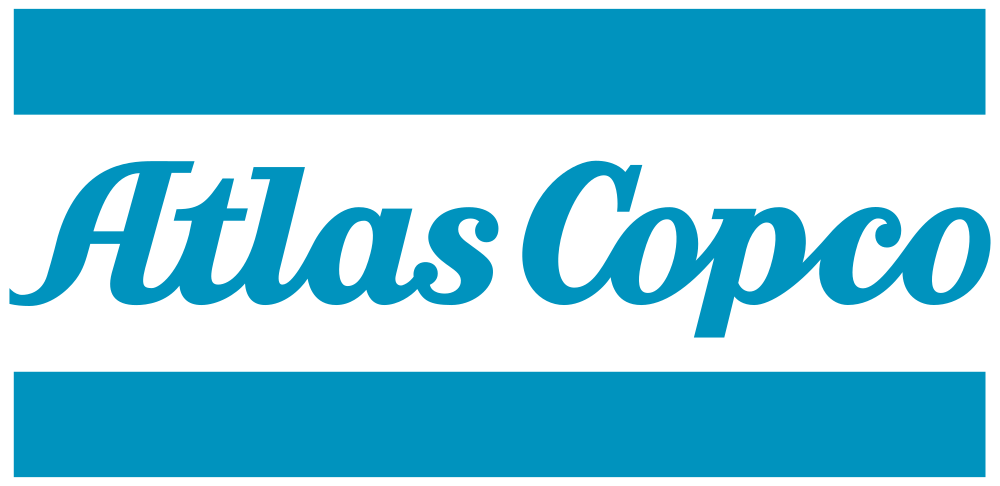
Challenges
The trays are arranged in 8m high, 3.5m deep and 3m wide container spaces. There are 8 containers in operation. Every single tray contains a different combination of parts. Manufacturing is guided by a weekly Manufacture Plan. Every day, the required parts need to be gathered from the trays and carried over to the production line, according to this Plan.
After getting to know the operation in depth, and several rounds of discussion, this has been the process we, together with the client, identified as one that could be improved with Machine Learning solutions. This would lead to higher efficiency and overall profitability.
Solution
Every single tray contains several types of parts. This offers us the opportunity of grouping those parts that will be needed together. This leads to less tray movement overall.
We optimized the process by two principles:
- We want the parts listed in the Manufacturing Plan grouped together on as few trays as possible.
- The 12 containers should be used similarly, to achieve a balanced workload on the personnel and the machinery.
It was clear from the start that when we get the next Manufacturing Plan, we can create instructions for a set number swaps (ie, ‘swap Type A parts from Tray 3, with Type F parts from Tray 11’), and the work requirement of these swaps, performed once a week, is lower than the value we get from the higher efficiency enabled by them.
This is a classic Machine Learning problem, which resembles the Travelling Salesman Problem. One of the possible mathematical solutions is ‘simulated annealing’ – this is a method which can solve the Rubik-cube as well.
Our product would be a software solution, which uses the weekly Manufacture Plan as starter data, and the endresult would be an e-mail received by the operators, that lists the 5 suggested swaps. The operators can perform these swaps before manufacturing starts on the new week.
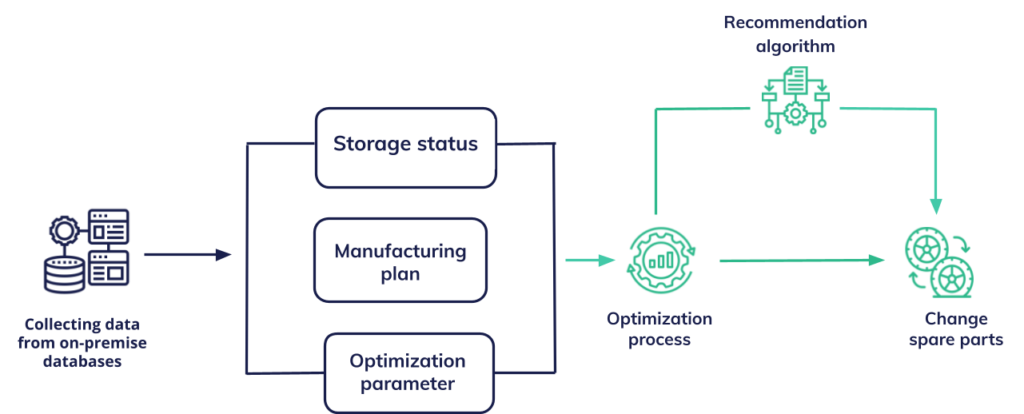
Results
The system has been tested and verified on legacy data.
We set up metrics which can be checked by the client on a custom Business Intelligence interface, realtime. One example would be: ‘How many parts are taken from the tray at the production line?’ This number moved upwards, since our objective was to minimize the tray movement time.
Case studies
Let's build something!
We’d like to hear more about you and what you have in mind.
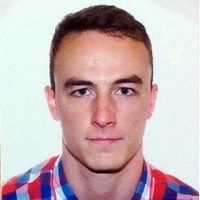
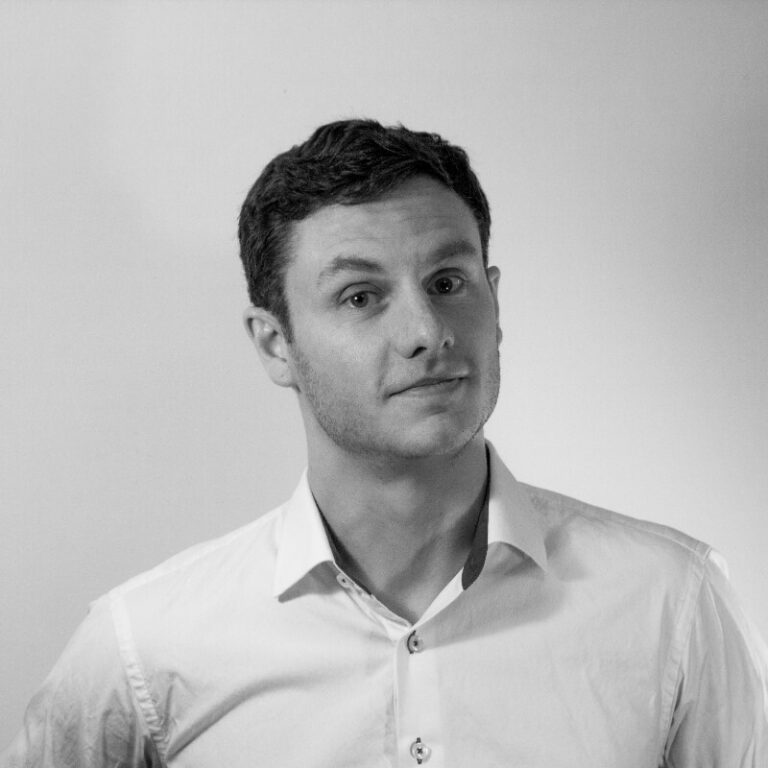
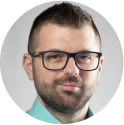
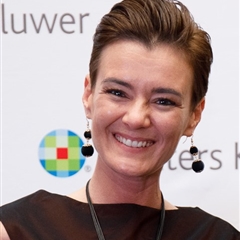
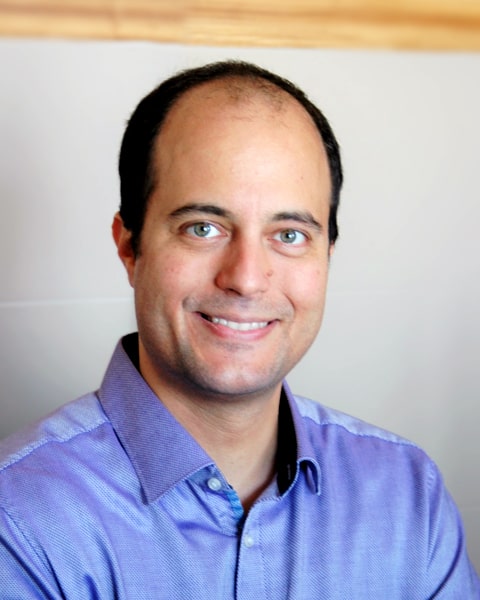